An all-optical associative learning element
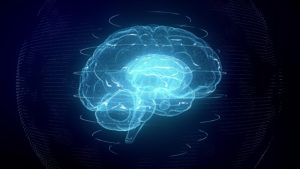
The interaction between humans and the environment and its impact on cognitive development has long been an inspiration in the development of autonomous systems and artificial intelligence (AI). Ian Pavlov’s classic experiment nearly one hundred years ago demonstrated the power of this type of learning by showing how dogs can be trained to associate a bell with food (the output being salivation). In recent years, there has been a drive to replicate such associative learning or ‘classical conditioning’ in the development of AI and machine learning technology.
Neuromorphic engineering, also known as neuromorphic computing, relates to the concept of using electronic circuitry to mimic neuro-biological architectures present within a human or animal nervous system. From a hardware perspective, the basic elements of neuromorphic computing, i.e., artificial neurons and synapses, have been devised. However, these elements do not form single-element associations but instead require an ensemble of such elements to associate between datasets, thus increasing the computational and energy requirements.
A new approach to all-optical associative learning
Building on previous work focussed towards developing an all-optical neuromorphic computer, researchers at the University of Oxford have devised an associative learning element. Such an element can be integrated with existing neuromorphic computing components to create circuitry which can receive inputs, capture associations and output reactions analogous to Pavlov’s dog experiment.
In this optical design, two complementary optical ‘sensor (input) neurons’ are embedded in two optical couplers. Energy (light pulses) from both couplers can be converged in an ‘optical motor neurone’ to determine when associations should be made. Simultaneous light pulses passing through each sensor neuron trigger a response in the ‘motor neuron’. This leads to different output behaviour of the motor neuron to capture the fact that an association has been made previously. When desirable, the learning element can be reset ready to ‘learn’ future associations.
Unlike existing software, the all-optical associative learning element developed at Oxford is completely embedded in simple hardware, which provides unprecedented design flexibility and scalability for future neuromorphic computers.
Commercialisation
This novel technology is the subject of a patent application. Oxford University Innovation is now seeking commercial partners to adopt the new technology and support its future development.
about this technology