Software to monitor battery health and predict faults before batteries fail
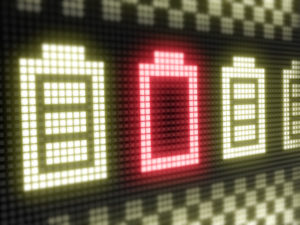
Importance of battery health
The UN Sustainable Development Goal 7 is to ensure universal access to affordable and clean energy. Yet despite progress in recent years, 770 million people still lack access to electricity according to the UN’s most recent estimates. Off-grid solar-battery systems play a vital role in addressing this global challenge; however, adoption is hindered by battery failure and rural locations that make preventative maintenance difficult.
Monitoring battery health is also mission critical in disaster relief, as well as the automotive sector, with battery failure being the most common cause of car breakdown.
While researchers have identified ways to monitor battery health in the lab, these techniques fail to work in the field, where it is difficult and costly to use the expensive equipment and high quality sensors that lab techniques require.
Oxford technology
Researchers at Oxford University have developed software to predict and diagnose faults in battery systems. The technology has been tested in the field and shown to work, allowing for battery health estimation that is robust to operating conditions.
The Oxford team has developed a scaleable probabilistic machine learning approach to diagnose battery health trajectories, which has been tested with 1,027 solar-connected lead-acid battery systems in sub-Saharan Africa. They have demonstrated accurate prediction of future battery failure, achieving a validated accuracy of 73% for forecasting faults eight weeks before they occur, rising to 82% at the point of failure.
This work uses a large amount of real operating data available across a fleet of batteries and highlights the opportunity to use cloud-based ‘big data’ techniques to understand battery health, extend the lifetime and improve performance in real-world applications.
The opportunity
The software provides battery health diagnosis and prognosis using only field data as inputs. This means there are no service interruptions or extra equipment required for this purpose. This is a big advantage, especially in mission critical applications and remote locations, where taking batteries out of service and individual testing is impractical. Further, timely diagnosis and prediction of faults allows for planning of maintenance and better logistics for replacements, as well as an improved understanding of stress factors affecting battery life.
The technology would be valuable for any company managing large fleets of batteries, for example, companies offering rural off-grid solar applications, energy grid storage, automotive OEMs and others.
about this technology