Sparse decomposition of arrays (SDA)
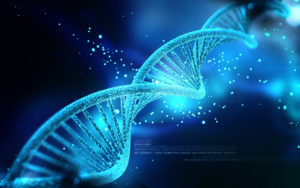
Genome-wide association studies (GWAS) aim to identify components of the human genome sequence that contribute to variations in physiological traits resulting from differences in gene expression. Identifying these components can help us better understand the molecular mechanisms underlying diseases and allow clinicians to formulate more appropriate therapeutic strategies. The data generated from GWAS can be difficult to interpret without the aid of sophisticated computational and statistical tools.
Oxford researchers have developed software using the Bayesian framework to decompose 3D arrays (or tensor) of multiple-tissue experiments to uncover gene networks linked to genetic variation. The software is validated by the application in the analysis of RNA sequencing data from 845 individuals from the TwinsUK cohort.
Genetic variability across individuals is responsible, to a certain extent, for the differences in physiological traits of an individual, including their susceptibility to diseases and response to drug treatments. Due to its clinical relevance, studies focused on discovering components of a genome that contribute to genetic variation have been widely conducted.
Gene expression traits
Expression quantitative trait loci (eQTLs) are loci that partly give rise to the variation in gene expression. eQTLs can operate proximally (cis-) or distantly (trans-) on a gene. So far, cis-eQTLs are easier to identify than trans-eQTLs. This is due to potential regulatory effects from the entire genome – as opposed to in the vicinity of a gene – is statistically and computationally difficult.
Oxford researchers have developed software that decomposes the tensor of gene expression datasets across multiple tissues and individuals in order to identify trans-eQTLs and gene networks that can lead to genetic variation based on a Bayesian method. The software confers the following advantages:
- Based on a novel and efficient algorithm
- Uses a flexible sparse assumption that can help uncover true, sparse underlying effects
- Complements current eQTL analysis pipelines that focus mainly on identifying cis-eQTLs in a single tissue
- Shown to work on real datasets (RNA sequencing data from 854 individuals from the TwinsUK cohort)
The work was published in Nature Genetics and the software is available online for academic usage.
Commercialisation
Oxford University Innovation is interested in hearing from organisations that would like to license this software commercially to support their research and development.
about this technology