Classification of electrocardiogram datasets
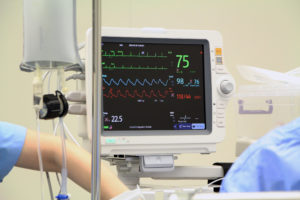
The rising prevalence of chronic diseases
One of the biggest global challenges we face is the delivery of healthcare to an ageing and expanding population. Chronic diseases are the leading cause of death for both developed and developing countries, representing 70% of all deaths, and cardiovascular disease (CVD) accounts for most of these (17.9 million annually). It is estimated that 85% of CVD deaths are due to heart attacks (i.e. myocardial infarctions) and strokes.
Traditional diagnosis of CVDs such as myocardial infarction mainly uses interpretation of electrocardiogram (ECG) recordings and blood tests, which requires precise acquisition devices and clinical expertise.
Diagnosis is difficult to achieve in a timely manner due to the slow generation of results from laboratory tests, as well as the inter-observer variability in ECG interpretation resulting in disagreement of diagnosis.
Putting AI at the heart of health care
There is a need to provide methods using machine learning algorithms with improved ability to classify ECG datasets, particularly for detection of heart disease.
Scientists at the University of Oxford have developed an improved and accurate method to classify ECG datasets. Applying these machine-learning algorithms to the combined datasets from multiple ECG leads means that correlations between the data from different leads can be taken advantage of to improve classification of the ECG dataset of the patient.
about this technology